On November 11, 2021, astronauts aboard the International Space Station repositioned to avoid an inactive Chinese satellite that was careening towards them and projected to pass within 600 meters of the station. Moves like this are becoming more common because of a specific culprit: space debris.
Space debris particles can be everything from an inactive satellite or a spent launch stage to metal fragments or paint flakes. While millions of undetectable smaller particles are believed to be orbiting earth, objects larger than a typical smartphone are tracked using ground-based telescopes. Over time, the increase of trackable particles is staggering: fifteen years ago, less than 10,000 space debris particles were being monitored this way. Today, it has risen 170 percent higher to over 25,000 particles, and continues to increase.
Moves to avoid debris like the November 2021 ISS example necessitate days of evaluation and, once approved, require hours to plan and execute. Until debris removal becomes a reality, governments and the commercial space industry are eager to improve the speed of detection in order to better facilitate avoidance.
Tackling latency issues is complicated and starts with ground-based monitoring. Current ground-based remote sensing, which incorporates a mix of radar, electro-optical, and laser technology, can be hindered by adverse weather, time of day, the material of the object being tracked, and the area each sensor can cover. These problems make accurate predictions on pathways and real-time reactions nearly impossible.
In 2021, Hyperspace Challenge worked with government officials who were eager to uncover technologies that could address these issues. As part of the fourth annual accelerator program, they asked cohort participants to conceive of technology that offers “smart sensing and machine learning for ground-based remote sensing of space objects.” Representatives from the Air Force Research Lab, along with a select group of startups and university teams poised to respond with applicable technologies, explored solutions through the program’s discovery workshops, one-on-one dialogues, and coaching.
Dr. Rajarathnam Chandramouli, after stumbling upon a LinkedIn post seeking submissions from universities, gathered a research group and applied to be part of the 2021 cohort. At the time, Dr. Chandramouli was a tenured professor of electrical and computer engineering at the Stevens Institute of Technology in New Jersey, and had spent several years developing machine learning algorithms for smart wireless and information systems. Indeed, for the 2019 Boston Marathon, he and his colleagues were invited to test their technology for resilient first responder wireless communications. With over 30,000 runners and over 100,000 spectators, signal interference and lack of consistent wireless coverage were high concerns for city officials. With the help of Dr. Chandramouli’s team communications signals remained strong throughout the event.
Based on that experience, Dr. Chandramouli and his colleague Dr. Subbalakshmi wondered if the same underlying algorithmic technology that proved to be successful on the streets of one of the busiest cities in the world, during one of its biggest public events, could be useful beyond the earth’s surface and adapted to support ground-based remote sensor tasking and data processing.
As part of the Hyperspace Challenge process, the team consisting of Dr. Chandramouli, Dr. Subbalakshmi, and Dr. Santhanakrishnan (New York Institute of Technology) met with government scientists in a series of discovery calls. During these sessions, they heard directly from the scientists about the challenges and issues they are facing.
“As it was described to us by the Air Force Research Lab, the problem right now is that space telescopes based on the ground are statically configured,” said Dr. Chandramouli. “So, if you have three telescopes, each looking at a certain region of the sky, they are going to be pre-programmed to only look at a specific area.”
But, as they learned, space debris can deviate from orbit for many reasons – from earth’s gravity and solar radiation to satellite emissions. If telescopes are statically configured, they don’t have an immediate way to move and evaluate deviations. Instead, they require human intervention. Dr. Chandramouli understood that this manual approach impedes rapid response.
Throughout the program, the team maintained a dialogue with government scientists. As conversations evolved, they learned the challenges extended beyond moving the telescopes themselves. “So much of the work is being done by intelligence analysts, trained personnel who look at data and imagery and then relay information to other teams asking for additional images or data,” said Dr. Chandramouli. All of these human touch points are opportunities for delay, and each could have real consequences if a collision was imminent. He added, “The question became, how can we make this process more efficient while maintaining accuracy and reinforcing the intelligence objectives.”
Dr. Chandramouli and his team proposed an algorithmic solution. As he describes, “our approach was an attempt to move from tactically configured states to dynamic ones.” He and his team started crafting an algorithm that prioritized dynamic optimization to respond to situational intelligence with the goal of tasking sensors using machine learning. This solution could minimize latency caused by manual readjusting.
Dr. Chandramouli believes these discovery sessions introduced a paradigm shift in his own thinking. Coming from academia, he was trained to think in long timelines with theories projecting out five years to several decades. This academic training also encouraged him to integrate assumptions in theories. A significant change in his thinking came from the conversations where government scientists proposed shorter timelines (sometimes months to a few years) and removed theoretical statements. “We have this academic training. We know how to solve problems, theoretically in most cases, at least in my field of engineering and computer science.” Dr. Chandramouli says. “What was really interesting to me,” he notes, “was when they explained the workflow, it left us with very little wiggle room to make assumptions. We might have said, ‘Let’s assume we have a million sensors,’ but the government scientists would say, ‘No, we have three.’” This openness and specificity directly supported the team in the development of their ideas.
Dr. Chandramouli is building on the momentum gained from the Hyperspace Challenge experience. In his current roles as a Principal Scientist at Galois and as CEO of Spectronn, an innovative wireless startup company providing transformative, cloud managed cognitive radio networking and mobile edge artificial intelligence technologies, he is focusing on the applications of the algorithms and finding new partners. His team is part of the 2022 Catalyst Accelerator and sees opportunities for developing the applications further. “I now have a personal goal of helping to reduce the latency in the cases we explored in the Hyperspace Challenge from days to thirty minutes or less by automating the whole process. There is satisfaction in solving, or at least trying to solve, a real problem. I’m not saying academics don’t solve real problems, but many times we address theoretical problems. In this case there is a very end-user-driven problem.”
To future participants in the Hyperspace Challenge program, Dr. Chandramouli offers these words of advice, “Come open minded and be ready to be challenged by the constraints presented in real-world situations. Your assumptions will be challenged by the discovery interviews. In many ways, that’s the most exciting part of the program.”
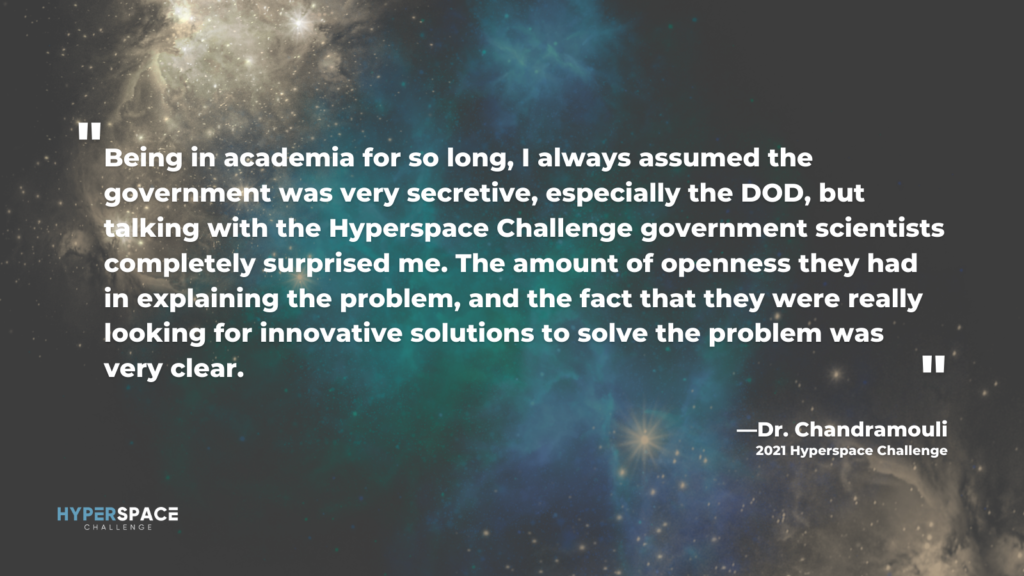